Unlock the full potential of your data: Transforming enterprise workflows with AI-powered data catalogs
Read the article below or download the PDF version here.
Modernizing data management for smarter insights and streamlined operations.
In today’s data-driven landscape, organizations struggle to manage their data assets effectively. Challenges range from centralizing information for easy access, ingesting data from numerous internal and external sources, automating end-to-end workflows and breaking down data silos and sharing data across departments. Without a streamlined approach to managing data and its metadata, organizations experience operational inefficiencies in accessing, curating and preparing their data to leverage AI, ML and modelling techniques to extract valuable insights and make informed decisions.
According to an IDC study on modern data management (September 2022), top management priorities for organizations include improving the collection, usage, and sharing of metadata, enhancing cloud migration strategies and automating key data management processes. However, achieving these goals requires comprehensive solutions that address data integration, storage, cataloguing, discovery, governance, and privacy, along with the development of internal and external data marketplaces.
AI-powered data catalogs are emerging as a critical component of modern enterprise data management strategies. These next-generation tools utilize Artificial Intelligence (AI) large language models and Machine Learning (ML) modelling techniques to automate metadata tagging, data discovery, and pattern recognition, enabling firms to more efficiently manage their data assets.
Although data catalogs offer significant advantages, their adoption is still in the early stages. A 2024 study by Dresner Advisory Services found that less than 20% of surveyed firms currently use data catalogs, despite over 80% considering them critical, very important, or important.
Enterprise Data Management Catalogs
Enterprise data catalogs serve as centralized platforms designed to help organizations manage, organize and govern their extensive and complex datasets. By offering a structured approach to cataloging and discovering data assets across various systems, such as databases, cloud platforms, and data lakes, catalogs make it easier to find, understand and leverage data.
The use of AI LLMs and ML techniques in catalogs speeds up data discovery by automating tasks like metadata tagging and anomaly detection, improving predictive analytics, and the provision of personalized data recommendations.
Growing Data Challenges
As organizations contend with the rapid growth of data sources, users, and use cases, they face significant challenges in managing their increasingly vast and complex datasets. Key issues include difficulty in discovering data hidden within siloed systems, inconsistent data quality, and the complexity of governance, especially when it comes to compliance and data security. Many organizations also struggle with data lineage tracking, collaboration across departments, and the efficient management of siloed data. AI-powered enterprise data catalogs are designed to address these challenges by improving data visibility, simplifying governance, and enabling scalable data management across the organization.
Example of problems and solutions addressed with an enterprise data catalog:
Problems
Solutions
Data Discovery Challenges
Data scattered across databases, data lakes, and cloud platforms makes it difficult for users to find relevant datasets for analysis.
Centralized Search Platform
Centralize all data assets into a unified, searchable platform, allowing users to easily find and access datasets.
Lack of Data Visibility and Accessibility
Organizations lack transparency about what data they have and who is using it, leading to underutilization of valuable data resources.
Improved Data Visibility
Provide a comprehensive view of data assets, metadata and usage statistics, making data accessible and visible to the organization.
Inconsistent or Poor Data Quality
Missing values, duplication or outdated data reduce the reliability of analytics and decision-making.
Data Quality Monitoring
Integrate tools for automated quality checks and notifications, ensuring high data standards and accuracy.
Data Governance Challenges
Managing governance, especially in large organizations, is complex, leading to security risks and regulatory non-compliance.
Governance Tools
Enforce governance policies, providing role-based access control, audit trails, and data lineage tracking to ensure compliance and security.
Lack of Data Lineage and Provenance
Without clear data lineage, users cannot trace the origin or transformations of data, undermining trust and replicability.
Data Lineage Tracking
Offer full visibility into the data lifecycle, from source through transformations, enabling users to trust and verify data accuracy.
Time-Consuming Data Preparation
Data professionals spend excessive time on data preparation and cleaning before analysis, delaying decision-making.
Streamlined Data Prep
Provide metadata, lineage, and quality metrics, reducing time spent on data preparation and allowing faster assessments.
Lack of Standardized Terminology and Data Definitions
Different teams interpret the same data differently due to inconsistent business terms and definitions.
Business Glossary
Offer a centralized glossary for standardized terminology and data definitions, ensuring consistent understanding across teams.
Compliance and Auditability
Increasing data privacy regulations make it challenging to track data usage and maintain compliance without clear audit trails.
Audit Trails and Compliance Tools
Catalogs provide robust audit trails, tracking how data is used, accessed, and shared, simplifying regulatory compliance.
A cloud-based data management platform like DataHex Data Library can drive organizations to insight and innovation through seamless centralized discovery, access and management of data workflow.
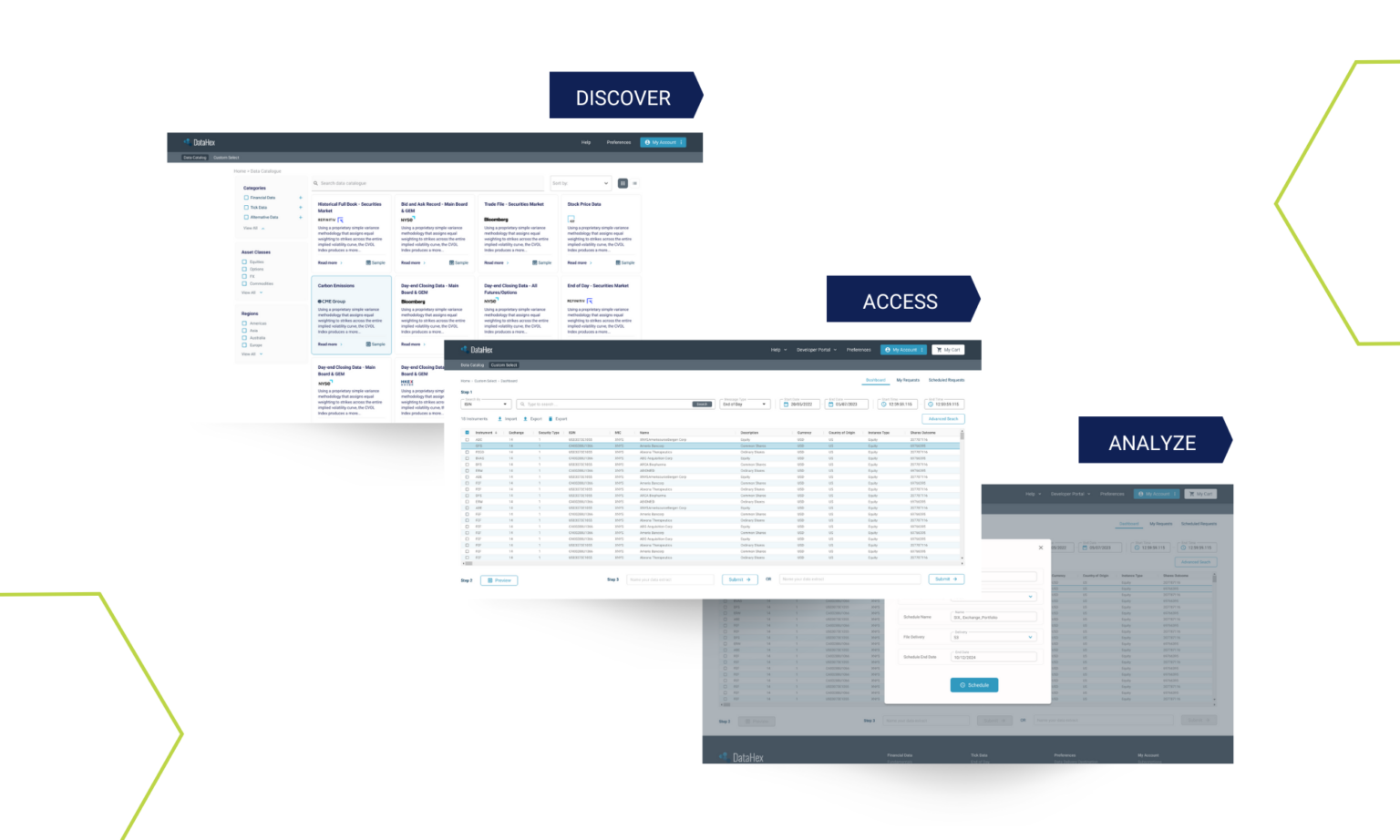
AI-powered Data Catalog vs. Traditional Data Management Systems
AI-powered data catalogs are advanced platforms that leverage artificial intelligence (AI) large language models and machine learning (ML) techniques to automate and enhance the process of organizing, discovering, and managing data.
Unlike traditional data management systems that rely heavily on manual processes for data classification, metadata tagging, and cataloging, AI-powered catalogs streamline these tasks by automatically tagging data, identifying relationships, and generating insights from datasets.
They go beyond simple data storage by offering intelligent data discovery, predictive analytics, and recommendations, making them more dynamic, scalable, and efficient than legacy systems.
Driving business value through:
- Enhanced Decision-Making: AI-powered data catalogs enable faster access to relevant and accurate data, helping business leaders make informed decisions and reduce the time to decisions. Automating the discovery and classification of data reduces the time spent searching for critical information. AI algorithms and models can quickly sift through large datasets, identify patterns, and suggest the most relevant data points, allowing decision-makers to access insights more efficiently.
- Reduced Time for Data Preparation: One of the most time-consuming aspects of traditional data management is the manual process of cleaning, organizing, and preparing data for analysis. AI-powered data catalogs significantly reduce this burden by automating tasks such as data cleansing, deduplication and normalization. Machine learning algorithms can detect and correct inconsistencies, remove redundant data and streamline data preparation, enabling data teams to focus on higher-value activities. Automating these processes enables organizations to accelerate data readiness for analysis, shortening the time from data ingestion to actionable insights.
- Automate Governance and Compliance: Governance and compliance are critical concerns for organizations managing large amounts of data. AI-powered data catalogs simplify governance by automatically enforcing policies and ensuring that data remains compliant with regulatory requirements. The systems can be enabled to detect sensitive information, apply appropriate access controls, and maintain an auditable trail of data usage and modifications. Embedding AI into governance workflows, organizations reduces the risk of non-compliance, improves data security, and maintains better control over data access and usage without manual intervention.
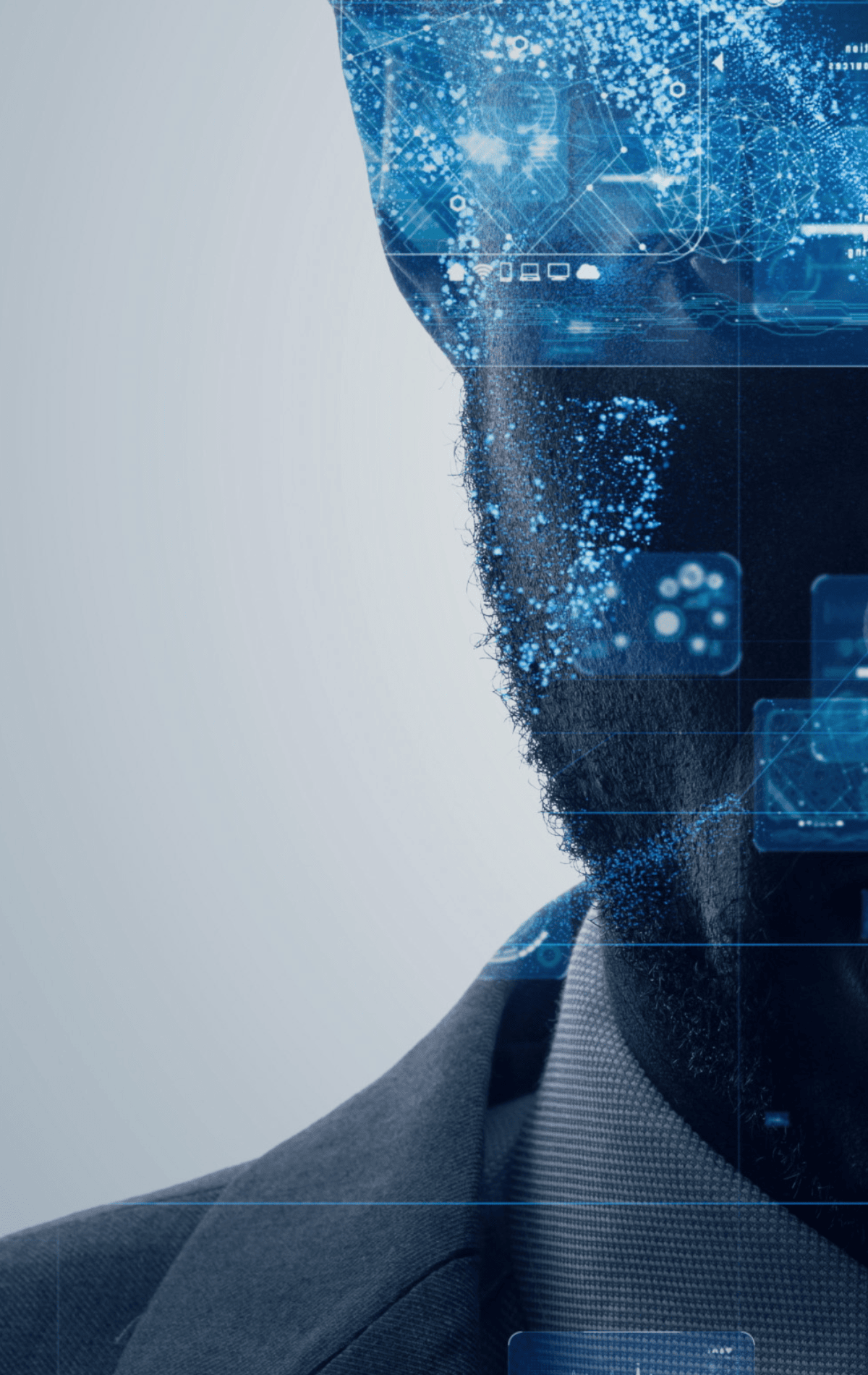

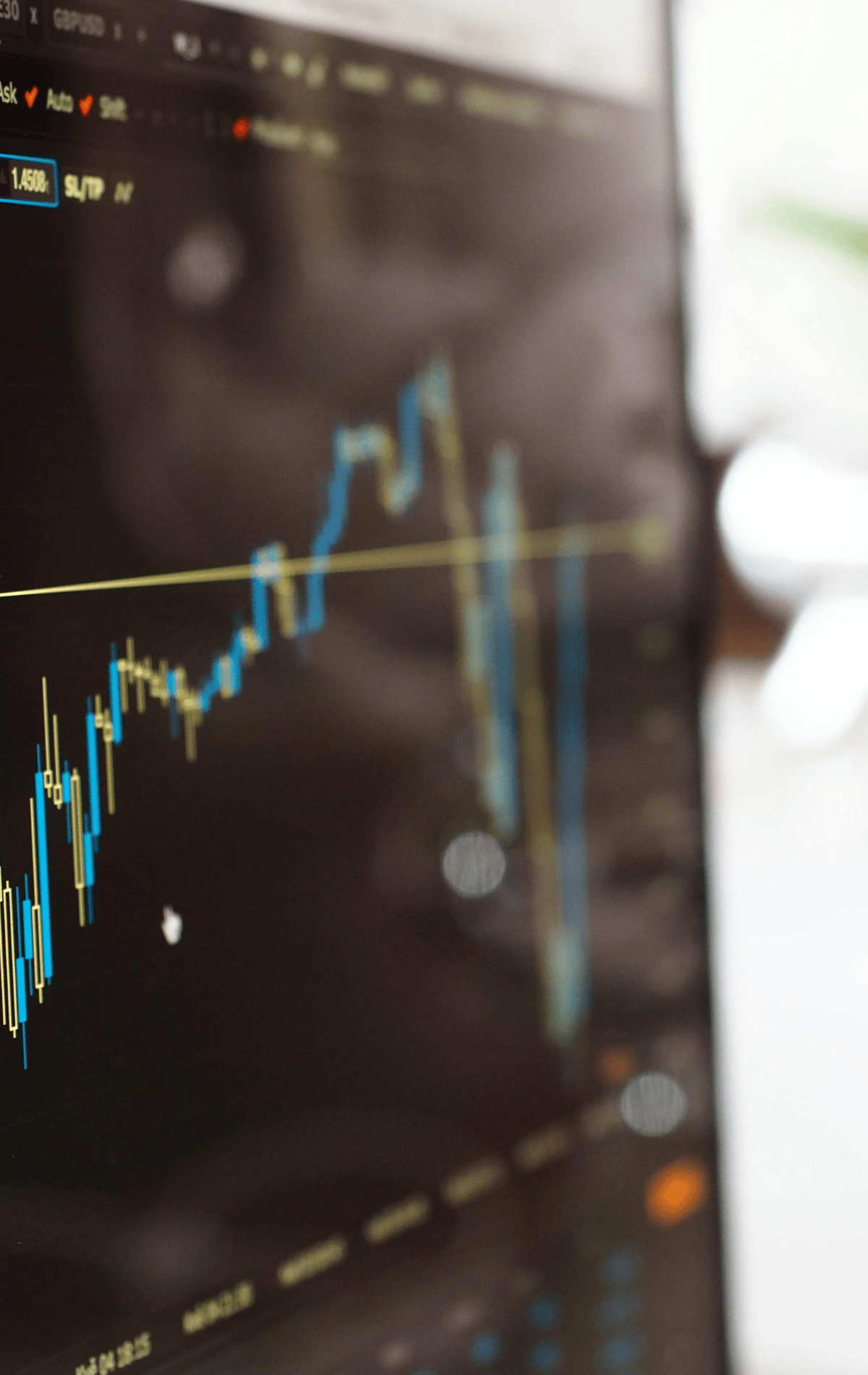
Key AI enhanced features in Data Catalogs:
- Automated Metadata Generation: AI-powered data catalogs automatically generate metadata by scanning and interpreting data assets from various sources. This reduces the manual effort needed to classify and organize data while ensuring that metadata is accurate, comprehensive, and up-to-date.
- Smart Tagging: Leveraging machine learning, smart tagging automatically assigns relevant tags to datasets based on their content. These intelligent tags enable easier searchability and classification, ensuring that data can be quickly found and utilized by different users within the organization.
- Intelligent Search: AI enhances search capabilities by understanding the context and semantics of search queries. Natural language processing (NLP) allows users to search for data using everyday language, while AI-driven language models and algorithms improve the precision of search results by accounting for user intent and contextual relevance.
- Data Lineage Tracking: AI can track the flow of data across systems, showing how data moves, transforms, and is used over time. This automated lineage tracking helps ensure transparency and auditability, which is crucial for governance and compliance purposes.
- Automated Data Quality Management: AI-driven catalogs continuously monitor data quality by detecting anomalies, errors, and inconsistencies. They provide automatic alerts and recommendations for corrective actions, helping organizations maintain high-quality data with minimal manual intervention.
Conclusion
AI-powered data catalogs are revolutionizing data management, providing smarter, more efficient ways to discover, organize, and govern enterprise data. By automating processes like metadata generation, data quality management, and governance enforcement, these tools enable faster insights and enhanced decision-making. As data challenges grow, adopting AI-driven catalogs is essential for businesses to stay competitive and unlock the full potential of their data assets.
About RoZetta Technology
RoZetta’s DataHex Data Library is more than a Data Catalog. It is a cloud-based platform that brings best practices to data management, analysis, and data science for Capital Markets. It centralizes access to both internal and external data, empowering users across the enterprise to quickly find data stored in multiple locations and spend less time wrangling data.
Drive your organization’s insight and innovation through seamless centralized discovery, access and management of your data workflow.
To learn more about how DataHex Data Library can drive your enterprise data innovation and insights, visit us at rozettatechnology.com or email us at enquiries@rozettatechnology.com
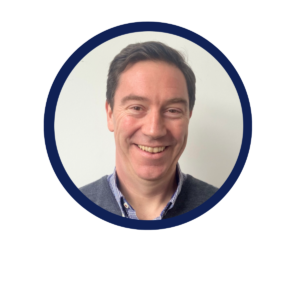
Peter Jones
Chief Product Officer, RoZetta Technology
Email: peter.jones@rozettatechnology.com
LinkedIn: www.linkedin.com/in/peterdysonjones/