How data strategy drives innovation
Read the article below or download the PDF version here.
Data-driven businesses prosper on good information with industry research across many market sectors revealing that a key driver of innovation is information. Information that is derived from data, enhanced, analyzed, integrated, and accessible through a core system.
This paper explores some common barriers to an optimal information strategy and opportunities that will drive innovation and increase profitability. The framework includes an Information, Analytics & Performance Platform and is consistent with using an enterprise-centric data management model.
Overview
Cross-industry studies show that, on average, less than half of an organization’s structured data is actively used in making decisions—and less than 1% of its unstructured data is analyzed or used at all. More than 70% of employees have access to data they should not, and 80% of analysts’ time is spent simply discovering and preparing data. Data breaches are common, rogue data sets propagate in silos, and companies’ data technology often isn’t up to the demands put on it.
Source: What is your data Strategy? Harvard Business Review
What are the most common barriers to innovation?
Which process improvements have the greatest potential to drive profitability?
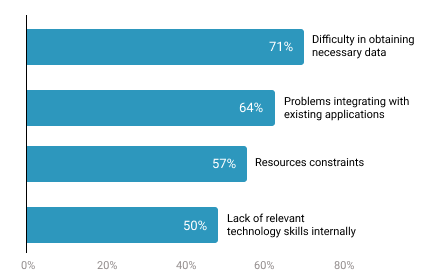
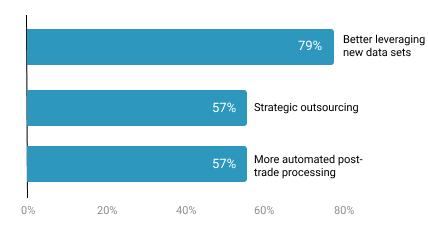
Source: Capital Markets Innovation: An Industry Conversation Finastra
Information Strategy
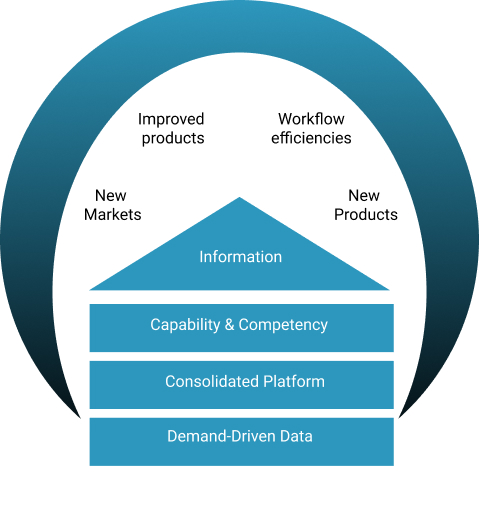
Figure. 1.1 Information drives innovation
Data is processed into a formal and familiar structure, with context, and enhanced with analytics to become information. Data refers to raw input that, when processed and integrated, becomes meaningful output: information.
Information should be accessible, relevant, enriched with analytics, and ready to use. Information solves problems, exploits opportunities and drives innovation.
Data Science has added new dimensions to information by efficiently interpreting unstructured text to identify entities and add to the depth of knowledge by applying sentiment, context, and entity associations. This capability has realized new insights and information from research, discussion forums, industry, and business publications.
An information strategy is crucial to align information management practices to meet governance requirements and inspire data users to explore new ways to attain the organization’s strategic goals and resolve the challenge of revenue and margin growth.
Supply Side or Demand-Driven Approach
The approach to developing an Information Strategy is a continuum of Supply Side to Demand Driven (Figure 1.2). The place on this continuum is related to the speed of innovation and the frequency of innovation required to build a competitive advantage.
A Supply Side, or centralized top-down approach, is often unsuited to supporting an effective information strategy (Figure 1.3). Supply Side focuses on controlling and standardizing an enterprise’s data; it tends to inhibit flexibility, making it harder to customize or transform data into information.
In a Demand Driven environment, end users exert far more influence on data acquisition and integration. A Demand Driven approach focuses on flexibility to resolve strategic questions, improve transactional outcomes, innovate new products, or increase workflow efficiency (Figure 1.3). Demand Driven information strategies support fast to discovery and fast to market.
For example, innovation in medicine heavily relies on long-term research and testing. It is concentrated on the Supply Side disciplines of control, exacting standards, and narrow interpretation, a necessary approach for medicine. Capital markets are at the opposite end with Demand Side traits of flexibility, short development times, often based on advanced analytics, and sometimes on intuitive leaps, with limited validation before going to market. Sometimes, being in the market is the validation. In capital markets, innovation is the key to outperforming competitors to attract new clients and retain existing relationships. In summary, Supply Side is about what is known, and Demand Driven is about what might be.
Figure. 1.2 Information strategy continuum
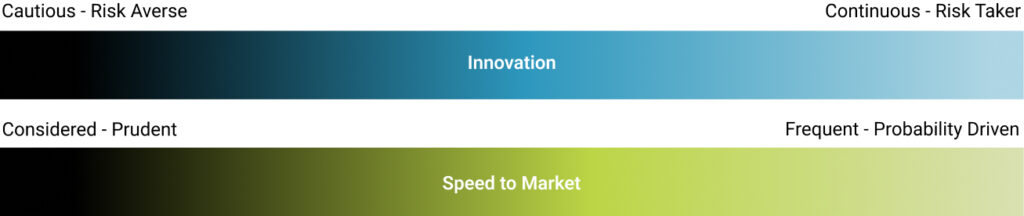
HEALTH
Innovation in health is the result of lengthy, meticulous research.
There are many regulatory hurdles to bringing innovation to market
MANUFACTURING PLANT
Developing large scale plant for manufacturing requires a long tail operation to recoup investment.
Frequency of purchase is low and lifecycle is long.
FINANCIAL SERVICES
Financial services, particularly business finance, is forced to constantly innovate.
This is driven by the increasing number of Fintech market entrants with new and creative products.
CAPITAL MARKETS
Innovation is often related to trading strategies.
The advantage of any single innovation is short lived as competitors replicate the strategy or new data becomes available to analyze and integrate.
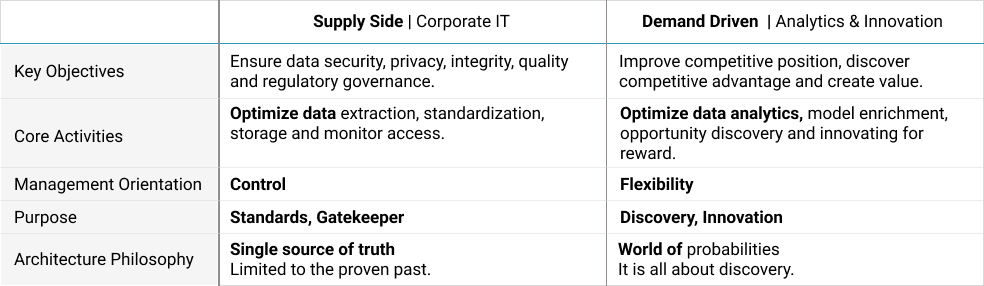
Figure. 1.3 Supply-side vs. Demand-driven comparison
Consolidated Information Analytics and Performance Platform
After deciding that a Demand Driven approach will succeed in driving growth, the next challenge is to visualize the information environment. The data, technology, capabilities, and competencies require seamless integration to ensure that information-driven decisions contribute to realizing key strategic imperatives.
The basic role of the consolidated Information Analytics and Performance Platform is to exploit technology, data, and technical capabilities to create a series of data assets that, when integrated, form an information layer to consistently support decisions related to achieving the organization's strategic imperatives.
Figure. 1.4 Information Analytics and Performance Platform
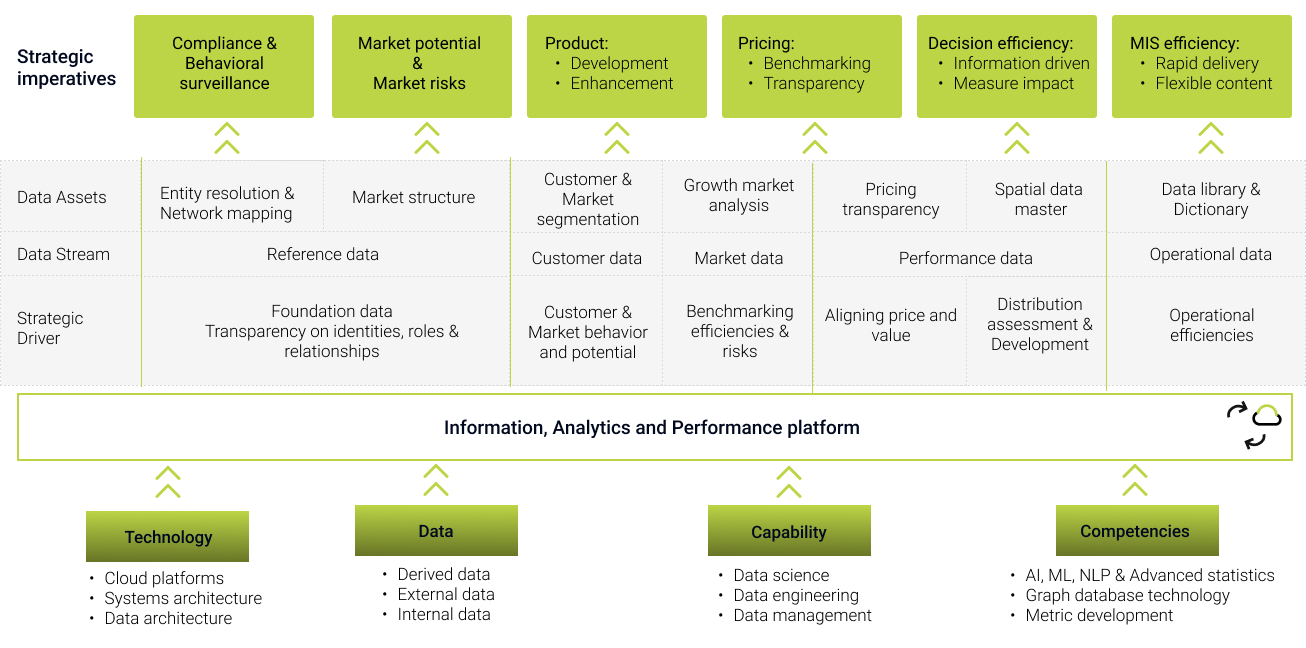
The Information Analytics and Performance Platform has three primary layers. (Figure 1.4) The first is incorporating the critical strategic drivers of the business's success. The next is the Data Stream, which identifies the type of data assets created; the final layer is establishing the data assets used by data engineers, data scientists, and quantitative analysts to derive innovations and efficiencies.
Following the structure left to right, it starts with developing high-quality reference data sets that identify all the external entities involved with the delivery and consumption organization’s products and services, including customers, intermediaries, suppliers, data vendors, and competitors. These relationships can be very complex, and using data engineering and data science skills, a graph database can capture these relationships and the strength, frequency, and monetary value of these relationships between entities. Using a graph database is particularly useful when monitoring the behavior across an intermediary network. It contributes to compliance and surveillance.
The next stage consolidates customer and market data. Different organizations will incorporate a range of data elements that feed into the segmentation of customers and markets.
For capital markets participants, this would include historical tick data. The analysis of these data sets would cover benchmarking to identify instances of deteriorating performance, overperformance, or probable inefficiencies.
These data assets also enable analysts to establish measures of potential and risk: the potential to grow and risk to shrink at customer and segment levels.
The development of the Information, Analytics & Performance Platform continues by incorporating and spatial data assets. Insights gained with these data assets include evaluating performance against the value proposition, aligning price against the cost to serve, measuring the value and relative performance of different channels, and identifying thin and thick markets by integrating market penetration, distribution effectiveness, revenue (current and potential), cost to serve, by segment and geography.
The last area in the Information, Analytics & Performance Platform is to add the organization’s performance or operational data.Developing these data assets enables the organization to discover areas of poor performance, inefficient procedures and highlight areas of probable waste.
The chapter - Enterprise Centric Model - later in this paper, demonstrates how implementing an Information, Analytics & Performance Platform and applying some basic principles go to the heart of the inefficiencies and waste in many reporting, analytics, and data science teams.
Capabilities and Competencies
Implementing a technical platform and data inputs is challenging and requires the right mix of capabilities and competencies. Each organization may require a slightly different complement of capabilities to optimize the value of establishing and evolving an Information, Analytics & Performance Platform. The statistical skills of senior quantitative analysts are often transferable to investigate and develop solutions in an adjacent industry, product, or function. However, this may not always be true and certainly isn’t with data scientists.
It is critical to spend time considering both capabilities and competencies that set up the Information, Analytics & Performance Platform for success.
Case Study
Proposition
A research company specializing in organizing round tables and seminars for global economic experts and recording and transcribing every event. This series of documents contained expert reviews and predictions on several topics. Sometimes about a specific commodity or financial future and often about predicted mergers and acquisitions.
The challenge is identifying all the subject entities in each forum, determining the topic applied to each entity, and then applying an identifier to enable matching back to listed entities.
Solution
Very specific data science skills were required to generate the output - entities, sentiment, context, and relationships, using advanced expertise in Natural Language Processing (NLP). The data scientist generated the outputs and passed them to a skilled data engineer who was required to achieve two goals. The first was to design how to capture within the existing data architecture, and the second was how to productionize the process/routine developed in Python to run seamlessly in the existing database environment as new transcripts arrived. Each entity then had industry identifiers attached to enable data interoperability.
An additional data source to augment the outputs and confirm the results, the process was expanded to incorporate global media feeds covering business, economics, and capital markets. Again having specialized data science skills linked to data engineering resulted in a continuing evolution of this as a data source for trading models. The process took a stream of qualitative data over time, matched it to market events, and established some leading indicators for a value or trading volume variation.
The case study shows the importance of having the right capabilities and competencies to produce the data output but also demonstrates how teaming up a data scientist and a data engineer created a new information set for the whole analytics team to investigate.
Enterprise-Centric Model
When ‘Enterprise Centric’ is used to describe a data management approach, it is not advocating an enterprise data management system. It proposes that an organization gains great value from having a single Information, Analytics & Performance Platform for analysts, data scientists, and reporting teams across the organization (Figure 1.5).
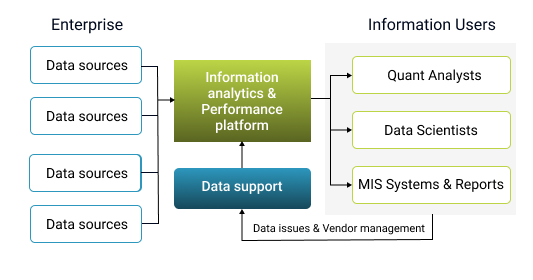
Figure. 1.5 Enterprise Centric Model
An Enterprise Centric platform, as opposed to User Centric, optimizes productivity and speed to decisions by presenting the best possible data to every end user in a format that is consumption ready, whether for developing trading models, discovery analytics, or reporting (Figure 1.6). Because remediation of all data enhancements and data quality issues are in the platform, the organization develops a unique data asset tuned to their specific needs, leading to achieving a competitive advantage through data.
An Enterprise Centric model has clear lines of accountability for acquisition, storage, normalization, completeness, data quality, vendor management, and data access.
Enterprise-Centric - Productivity increases by socializing benefits
Figure. 1.6 Benefits of Enterprise-Centric Model
- License data once
- Data quality issues fixed for all users
- Multiple entity identifiers enable easier testing & integration of alternate data
- Catalog function for discovery
- Data enhancements benefit all users
- Single point of contact for data vendor issues
Summary
Implementing an Information, Analytics & Performance Platform will overcome the key obstacles to innovation reported in Finastra’s research.
The underutilization of data highlighted in the HBR report is also addressed.
In addition to providing a platform for innovation, implementing an Information, Analytics & Performance Platform delivers a really high cost saving over having a very distributed model for acquiring and managing data for analysis and reporting. While it does not allow an organization to remove all the costs associated with data wrangling, the experience is that the current cost base of data wrangling and data quality downtime is reduced by ~50%.
The Information, Analytics & Performance Platform allows skilled analysts and data scientists to be far more productive, and creates a higher level of innovation and value creation.
About DataHex
RoZetta’s DataHex will deliver a turn-key infrastructure bringing best practices to data management, analysis, and data science utilizing cloud technology. The solution ensures clients retain control of their data and ownership of insight, innovation, and IP that comes through easy access to data sets, tools, and services.
DataHex SaaS Platform provides -
- Scalable, repeatable, purpose-built cloud platform and managed service specialized for data analysts, data scientists, and managers of data-dependent workflow.
- Proven platform that de-risks and eliminates bottlenecks in managing large-scale time series data.
- Streamlined data access via a search engine, data mapping, API connectivity, and cloud delivery, enhancing the overall consumer experience.
- Powerful analytic discovery enabling more Data Science time to be spent on analysis rather than cleansing and preparing raw data.
Why RoZetta Technology?
RoZetta Technology believes that fusing data science, technology, and data management is the path to amplifying human experience and knowledge.
Our clients have a deep understanding of the challenges they face. We bring proven capability, experience and a mindset to create products and systems that overcome these challenges and create more value while solving them. No matter how complex, the blend of good data, the right technology, well-crafted design and smart individuals can solve most problems.